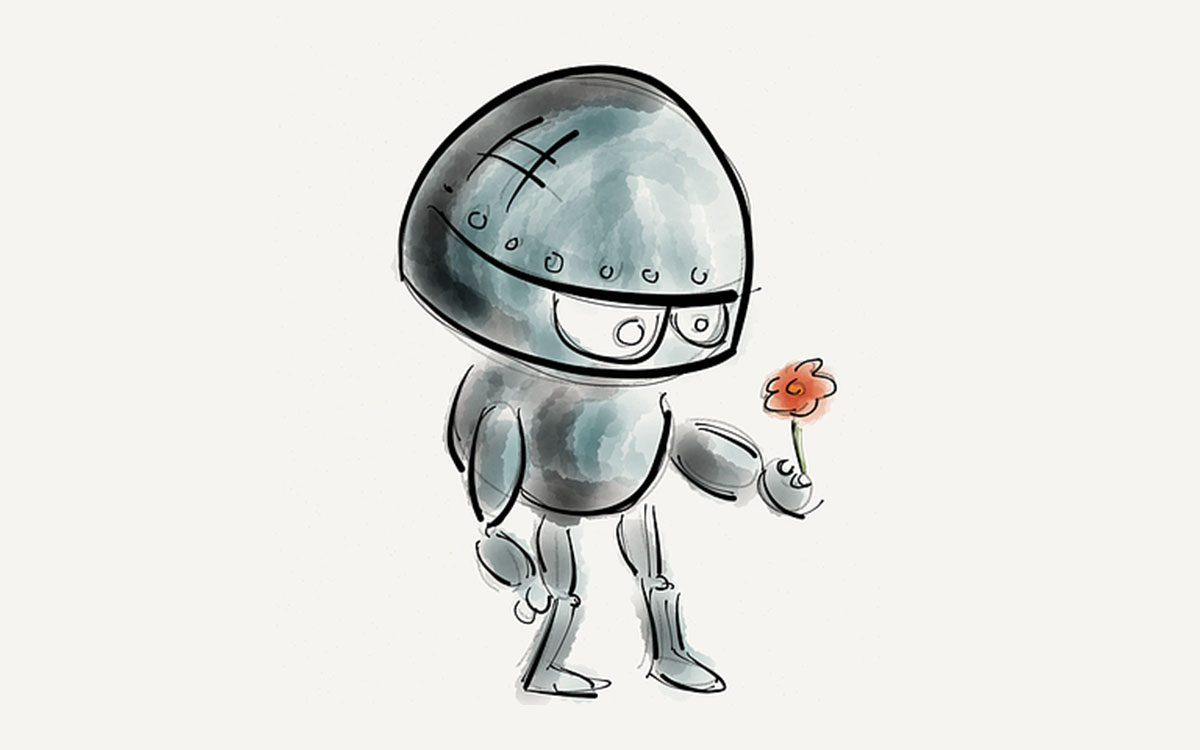
Civilization advances by extending the number of important operations we can perform without thinking about them. – Alfred North Whitehead
Whether we realize or not, Machine Learning and Artificial Intelligence are all around us in every bit of our life. The cars we drive or the news we read or the product recommendations we receive in our eCommerce site or the videos we watch or the search results we consume or the posts you read in your social media platform, all are driven by machine learning algorithms of some form.
Slowly, but surely, the same algorithms are making their way into the workplace as well, replacing one job after the other from customer help desks to factory floors to financial advisors.
Much has been talked about these days on the effect of machines and automation, from the perspective of the social impact related to the very survival of the coming generation. More automation, jobless economic growth, fewer jobs for the younger generation and where would the purchasing power come from?
Here is an interesting tale in the context of automation – a conversation between Henry Ford II and Walter Reuther, the leader of the United Automobile Workers.
Henry Ford: “Walter, how are you going to get those robots to pay your union dues”
Reuther replies, “Henry, how are you going to get them to buy your cars?”
Hmmm. Interesting conundrum!
Recently I came across this interesting research paper, on ‘When will AI exceed Human Performance?’ from the more appropriately named ‘Future of Humanity Institute‘ at the Oxford University.
This research paper is an inference from the survey response of some 350+ academics and industry experts in machine learning. This research study defines Automation as below:
When are all occupations fully automatable? That is, when for any occupation, machines could be built to carry out the task better and more cheaply than human workers.
As the research paper alludes to, developments in AI and Machine Learning has more social implications than the sheer fascination of these technology advancements. Autonomous cars, Amazon Go, Google Assistant, Tesla Gigafactory, all can potentially eliminate thousands of jobs and they have already done so.
What fascinated me in this study was the attempt to predict when would the machines really take over the Humans in thinking.
Perhaps the moment in the evolution cycle that we fear, that happened to the Primates could potentially happen to us, humans.
As Nick Bostrom writes in Superintellgence,
As the fate of the gorillas now depends more on us humans than on the gorillas themselves, so the fate of our species then would come to depend on the actions of the machines intelligence.
But, we have one advantage over the gorillas: we get to make the first move with machine intelligence. We write the code for the initial algorithms or we create the initial training datasets. How soon will it be before the machines become good to engineer the initial conditions as well and build on top of it? How soon would the machines really become good at creating things – for themselves?
Here are some of the findings which I found interesting, especially given the diversity of the responses by demographics:
The overall prediction is that there is a 50 percent chance that AI will be better than humans in about 45 years. This number ’45 years’ is the median response from all the experts.
Asians (read as the most populous, and most vulnerable to the real threat of job losses for their young population) see a higher probability of automation of every job, much sooner than the North Americans. They see the AI takeover coming in just 30 years compared to 74 years, as predicted by the Americans. Is this primarily driven more out of the ominous job threats for the younger generation?
While there is no question on the machines taking over ‘rule based’ tasks, what’s interesting is the prediction that machines would be better at some creative tasks like ‘writing essays and songs’. There is even a prediction that AI should be writing New York Times Bestsellers in 33 years!
So, it’s all about probability and predictions on when Machines would really take charge, based on questions posted to humans.
I wonder, how would a Machine Learning algorithm answer this question based on what they do today? Or, would it even be possible for it to answer this question?
Perhaps, there lies the answer to what is automatable and what is not.