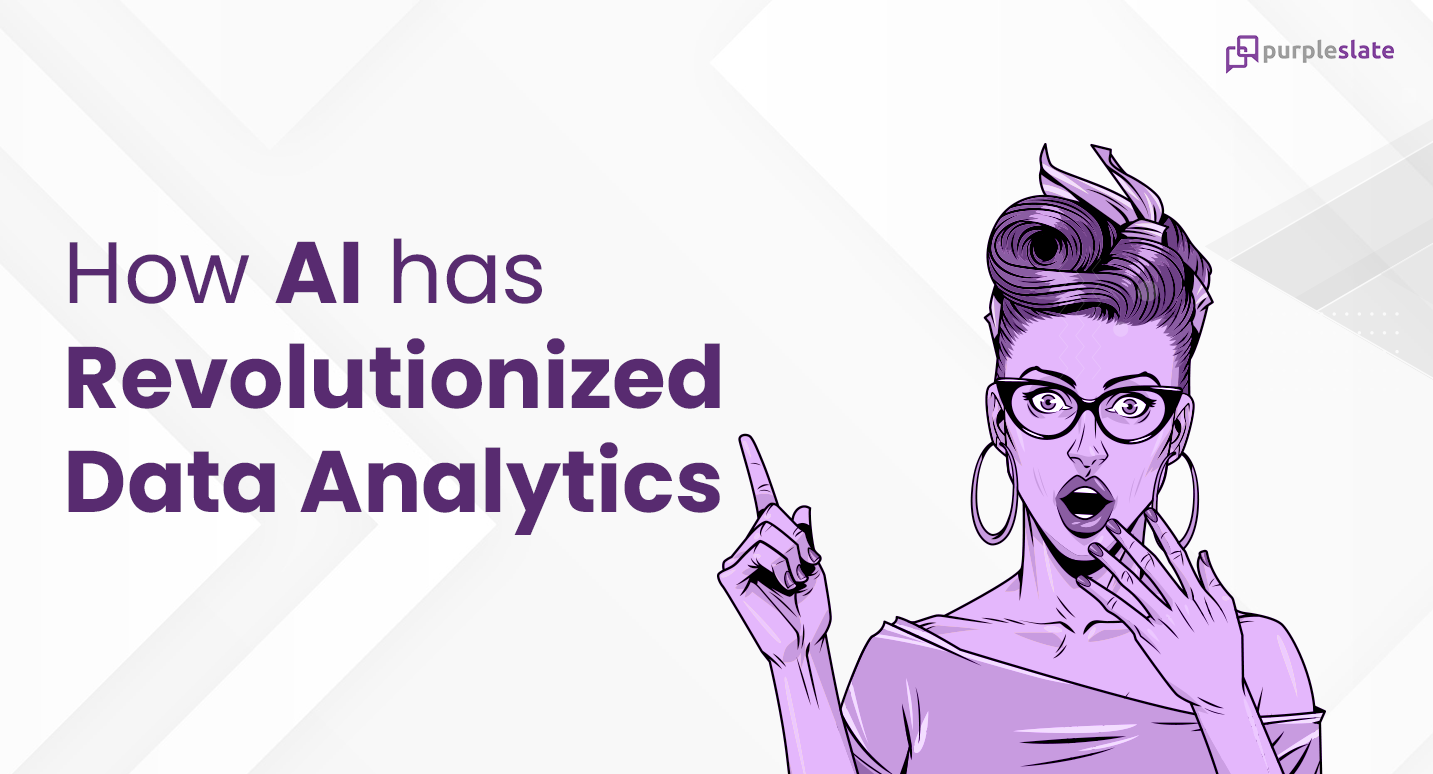
Introduction
Data analytics has changed the way we approach business. It has helped in making better decisions, which in turn have benefited us immensely as individuals and organizations. Data is considered a valuable asset by large organizations and they invest in acquiring more of it to gain a competitive edge.
Data analytics is a field that has been revolutionized by Artificial Intelligence (AI) since its inception. AI has helped in improving the quality of data analytics and reducing the time required to analyze it. AI has made it easier for businesses to analyze complex data sets, unstructured text-based information such as social media posts or web pages, and even different sources of information at once.
What are the Different Types of Data?
The first step is understanding the different types of data that AI ingests to work its magic. Data can be classified into three main types: structured, unstructured, and semi-structured.
- Structured data is organized and labeled; it contains attributes that are represented in a hierarchical or tabular manner. E.g., students’ names, employee details, etc.
- Unstructured data is not organized or labeled; it consists mostly of text files such as documents, emails, etc., but also audio recordings like telephone conversations or video files such as movies or TV series episodes.
- Semi-structured information includes structured information where some relationships have been identified between its elements, but there’s no additional metadata attached to them yet.
Augmenting Data Analytics with Artificial Intelligence
By embedding or combining AI and ML technologies with data analytics and business intelligence (BI) tools, organizations should be able to tackle the most complex data types and uncover the hidden value of unstructured data at scale – Forbes
AI has also evolved into an effective tool for learning from past experiences and anticipating future outcomes. It’s being used to predict future outcomes, make decisions, find patterns in data, make sense of data, and perform tasks that are too complex for humans to manage alone. This technology is driving innovation and improving business outcomes by helping companies make better decisions. One example is the insurance industry, where AI-powered data analytics helps improve customer service and save money. Another example is in the retail industry, where it helps predict full-price sell-through, gives in-depth insights as to when to order the next batch, and forecasts customer footfalls in a store-wise fashion.
A wide range of tasks can be accomplished through AI-based analytics, including data cleaning and transformation, model building and selection, predictive modeling, and recommendation engines. In particular, AI is well suited for data management and cleansing tasks such as feature extraction, entity generation, and classification. The speed at which an algorithm learns from its environment makes it possible for many types of problems that were previously infeasible to be solved by AI in a very short period.
There are majorly two areas that AI-driven data analytics enhances for decision-making.
Speed of Decision Making
AI models can immediately and accurately identify any changes in the forecast. Add to this package, the clustering and correlation algorithm that helps in root cause analysis. The biggest advantage that comes with AI-driven models is the real-time analysis as opposed to the quarterly, and monthly sessions, which will speed up decision-making considerably.
Automation at Scale
AI can proactively search for anomalies – data points that deviate from the dataset’s normal behavior. It does that by continuous learning based on the datasets it has crunched. Detecting and flagging anomalies is critical as it can help make potential changes in the management decision. For example, in a customer dataset, if the demography of the target group has changed and AI flags it, relevant changes can be made to the customer acquisition strategy. Automating this with little to no supervision ensures that more thought can be put into the decision, and AI can repeat this exercise with great levels of granularity.
Real-Life Impact of Implementing AI-Driven Analytics
There are many real-life use cases for implementing AI in data analytics. AI ensures speed to decision-making while accurately identifying patterns or anomalies, along with automating these detections at scale. These activities have far-reaching impacts across the length and breadth of different business functions. For the sake of this blog, however, we will limit it to three use cases.
- Demand Forecasting: A very well-known and well-implemented use case of AI-driven analytics is demand forecasting. This is of prime importance in sales and supply chain as their entire year’s order movement will be heavily dependent on these numbers. Getting the forecast as close to perfect as possible can be a lifesaver in optimizing operational overheads.
- Predictive Maintenance: There are two different maintenance techniques when it comes to machines on the shop floor. One is called breakdown maintenance, where the aim is to make sure the machine that broke down is up and running in no time. The other one is preventive maintenance where frequent maintenance is carried out to reduce the chance of breakdowns. With AI-powered data analytics, possibilities of breakdowns can be predicted earlier and preventive maintenance frequency can be increased or decreased. Additionally, if the machine needs replacement, or certain spares are going to expire, it can be proactively predicted by AI.
- Customer Behavior: This is another core use case where AI-driven data analytics come into play. Today, the data collection and storage for a customer’s buying behavior has grown exponentially and companies are sitting on a gold mine of customer data. Understanding customer behavior will help organizations plan well in advance right from product design to channels of sales. AI tools can easily identify changes in buying behavior and flag them in advance to plan for strategy changes. Additionally, they can also help organizations predict changes in tastes and preferences based on culture, politics, available technology, target generation, geographics, and more.
Parting Notes
The world has gone from where people had to rely on their judgment and intuition, to where machines can do most of the work for us. In many cases, these algorithms have been able to aid humans to make better decisions and they are improving at an exponential rate. With all this in mind, we can say with confidence that AI will become ever more prevalent in data analytics.
But does the evolution of data analytics settle down with introducing AI on its bandwagon? We feel this is but the start of a new era in data analytics and business intelligence, one which metamorphosizes to become conversational. Intrigued by the thought? Let us introduce you to the next generation of data analytics aided by conversational AI – Conversational insights. And, this is the right time to embrace it.
Check out our infographic on how the world is slowly moving towards conversational insights.